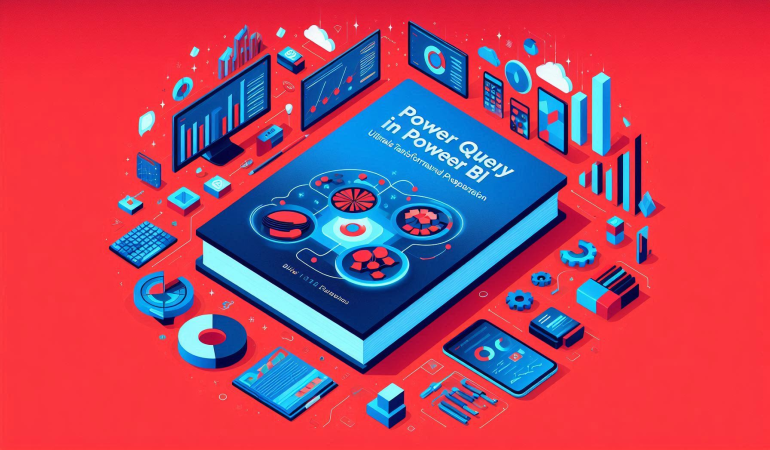
Power Query in Power BI: Ultimate Guide to Data Transformation and Preparation
Oct 31, 2024
Power Query in Power BI: Ultimate Guide to Data Transformation and Preparation
Introduction
In today’s data-driven world, the ability to transform and prepare data for analysis is invaluable. Power Query in Power BI offers a robust set of tools that allows analysts, data scientists, and business users to connect, transform, and visualize data efficiently. This ultimate guide dives deep into the Power Query tool within Power BI, providing a comprehensive overview of its features, capabilities, and how it can revolutionize data transformation and preparation for various projects.
Table of Contents
- What is Power Query in Power BI?
- Why Use Power Query?
- Understanding the Power Query Editor
- Connecting Data Sources
- Data Transformation Basics
- Applying Transformations with Power Query
- Cleaning Data with Power Query
- Shaping Data for Analysis
- Advanced Transformations
- Creating Custom Columns and Measures
- Using M Language in Power Query
- Power Query Best Practices
- Common Use Cases for Power Query
- Limitations of Power Query
- Power Query vs. DAX: When to Use Which
- Power Query FAQs
1. What is Power Query in Power BI?
Power Query is a data connection and transformation tool integrated within Microsoft Power BI, Excel, and other platforms. It enables users to connect to diverse data sources, shape and transform raw data, and make it ready for analysis. Power Query supports a wide range of file formats, databases, and online services, providing a bridge between data silos and the analytical capabilities of Power BI.
2. Why Use Power Query?
Power Query streamlines data preparation, one of the most time-consuming parts of the data analysis process. Instead of manually handling data cleansing and transformation, Power Query offers a no-code solution with an intuitive interface. It allows users to automate repetitive data preparation tasks, improves data accuracy, and enhances workflow efficiency. For anyone looking to make data-driven decisions, Power Query reduces complexities and ensures data is prepared, consistent, and ready for advanced analytics in Power BI.
3. Understanding the Power Query Editor
The Power Query Editor is the workspace where all data transformation and preparation actions are carried out. It provides a user-friendly environment with a variety of functionalities to manipulate data without any coding. The Power Query Editor includes:
- Query Pane: Displays all active queries.
- Data Preview: Shows a sample of the data from the active query.
- Ribbon Toolbar: Offers various transformation commands.
- Applied Steps Pane: Lists each transformation applied to the query, enabling users to track and modify their data transformation journey.
4. Connecting Data Sources
Power Query can connect to a broad spectrum of data sources, including:
- Local Files (e.g., Excel, CSV, Text)
- Databases (e.g., SQL Server, MySQL, Oracle)
- Online Services (e.g., Microsoft Azure, Salesforce)
- Web Pages This range of connectivity ensures that users can work with data from multiple origins, making Power Query a versatile tool for diverse data preparation tasks.
5. Data Transformation Basics
Data transformation in Power Query involves changing data from its raw format into a structured format suitable for analysis. Key transformation tasks include:
- Renaming columns
- Changing data types
- Filtering rows
- Removing or replacing values
These transformations are stored as “steps” within Power Query, ensuring a reproducible data transformation process that can be modified or extended as needed.
6. Applying Transformations with Power Query
Power Query provides various transformation options, accessible from the toolbar, including:
- Transpose: Switch rows to columns and vice versa.
- Pivot and Unpivot: Convert rows to columns and columns to rows, often used in restructuring data tables.
- Group By: Aggregate data based on specified columns.
- Merge Queries: Combine data from two tables based on a matching key.
Each of these transformations can be easily applied and viewed in real time, allowing users to experiment with different data setups until they find the ideal format for analysis.
7. Cleaning Data with Power Query
Data preparation involves substantial data cleaning, as raw data may contain inconsistencies, missing values, or duplicates. Power Query offers essential tools to clean data, including:
- Remove Duplicates: Eliminate duplicate rows based on specified columns.
- Remove Errors: Clean up data by removing rows with errors.
- Fill Down/Up: Populate blank cells in a column by filling down or up.
- Replace Values: Replace specific values in the data set to correct inconsistencies.
These features make Power Query an invaluable tool for preparing clean and reliable data, which is essential for producing accurate insights.
8. Shaping Data for Analysis
Shaping data involves altering the structure of your data to fit analytical needs. Power Query enables users to:
- Reshape columns and rows
- Split and merge columns
- Extract specific data segments
Shaping data ensures that it aligns with the required schema or format for analysis, making it easier to visualize and interpret in Power BI.
9. Advanced Transformations
Power Query also supports advanced transformations to accommodate more complex data preparation needs:
- Conditional Columns: Create new columns based on conditional logic.
- Custom Columns: Define columns with custom formulas for specialized calculations.
- Appending Queries: Stack data tables with similar structures to unify multiple data sources.
Advanced transformations can be especially helpful in handling large datasets with multifaceted data structures or intricate preparation requirements.
10. Creating Custom Columns and Measures
Custom columns and measures allow users to perform tailored calculations and transformations within Power Query. Some common examples include:
- Concatenation: Combine multiple columns into one.
- Mathematical Calculations: Perform addition, subtraction, and other arithmetic operations.
- Text Manipulation: Extract or transform text data within columns. Custom columns and measures add flexibility, enabling users to shape their data precisely to fit analytical objectives.
11. Using M Language in Power Query
M Language is the powerful scripting language behind Power Query. While users can perform most tasks with the GUI, M Language allows more granular control for complex transformations. Some benefits of using M Language include:
- Custom Function Creation: Write reusable functions for specific transformation tasks.
- Advanced Querying: Build complex queries that go beyond GUI capabilities.
- Performance Optimization: M Language can streamline code, enhancing query performance for large datasets.
For users comfortable with coding, M Language expands Power Query’s functionality significantly, enabling highly customized and efficient data transformations.
12. Power Query Best Practices
Adopting best practices ensures efficient and maintainable Power Query workflows:
- Organize Queries and Steps: Name queries and steps descriptively for better traceability.
- Remove Unused Columns: Minimize data load by removing unnecessary columns early in the process.
- Limit Transformations in M Language: Where possible, use built-in transformations as they are optimized for performance.
- Document Transformation Steps: Adding comments helps collaborators understand each transformation.
Implementing these practices streamlines data preparation and helps avoid performance issues or confusion within complex projects.
13. Common Use Cases for Power Query
Power Query is applicable across a variety of data transformation scenarios:
- Data Consolidation: Combine multiple data sources into a single table.
- Data Cleansing: Standardize formats, remove duplicates, and correct errors.
- Report Preparation: Shape data to fit report specifications.
- Data Integration: Merge data from different sources for comprehensive analysis.
These use cases highlight Power Query’s versatility in various domains, from business intelligence to financial reporting and scientific research.
14. Limitations of Power Query
While Power Query is powerful, it has some limitations:
- Large Data Volumes: For very large datasets, performance can be slower.
- Limited Visualization: Power Query is designed for transformation, not visualization.
- Learning Curve: M Language can be challenging for beginners.
Despite these limitations, Power Query remains a robust solution for most data preparation tasks, particularly within the Power BI ecosystem.
15. Power Query vs. DAX: When to Use Which
Power Query and DAX (Data Analysis Expressions) serve different roles in Power BI:
- Power Query is ideal for initial data preparation, cleaning, and shaping.
- DAX is best for analytical and calculation-focused transformations after data is imported into Power BI.
A hybrid approach often works best, using Power Query for data preparation and DAX for in-depth analysis and complex measures.
16. Power Query FAQs
What is Power Query mainly used for in Power BI?
Power Query is primarily used for connecting, transforming, and preparing data from various sources, making it ready for analysis within Power BI.
Can Power Query handle large datasets?
Yes, but performance may decrease with very large datasets. Optimizing query steps and minimizing unnecessary transformations can help improve performance.
Do I need to learn M Language to use Power Query effectively?
No, M Language is optional and primarily for advanced transformations. Most tasks can be completed using the GUI interface in Power Query Editor.
What is the difference between Power Query and DAX?
Power Query is used for data preparation (cleaning, shaping), while DAX is used for data analysis and calculations within Power BI once data is loaded.
Can I undo transformations in Power Query?
Yes, each transformation step is recorded in the Applied Steps pane, allowing you to delete or edit previous steps without starting over.
How do I connect Power Query to different data sources?
In Power BI, open Power Query and select “Get Data” to choose from the wide range of available data sources, including files, databases, and online services.
Conclusion
Power Query is a fundamental tool in Power BI, empowering users with the ability to seamlessly connect, transform, and prepare data for insightful analysis. By following best practices, understanding Power Query’s extensive features, and leveraging the power of M Language, users can efficiently handle complex data transformation tasks. Whether you're a seasoned data analyst or new to Power BI, mastering Power Query opens up vast opportunities to streamline data preparation and enhance the analytical potential of your projects.
For more detailed guidance and in-depth training, visit our training here.