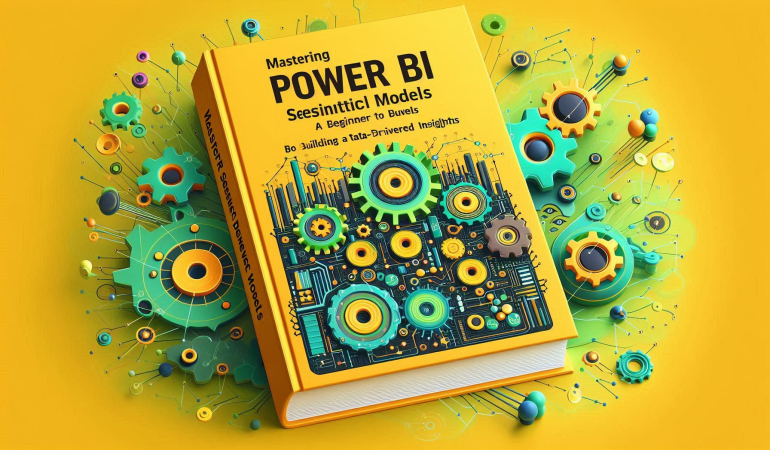
Mastering Power BI Semantic Models: A Beginner's Guide to Building Data-Driven Insights
Oct 27, 2024
Mastering Power BI Semantic Models: A Beginner's Guide to Building Data-Driven Insights
The growing importance of data analytics has put tools like Power BI at the forefront of business intelligence and data visualization. A key component in Power BI is its semantic model, which serves as the foundation for creating robust, data-driven insights. Semantic models in Power BI act as a bridge between raw data and meaningful analysis, enabling users to create visualizations and reports that help in making informed decisions.
In this beginner's guide, we’ll explore what semantic models are, how to create them in Power BI, and how mastering them can elevate your data analytics capabilities.
Table of Contents
- What is a Semantic Model in Power BI?
- Why Use Semantic Models?
- Understanding Data Modeling Basics in Power BI
- Data Preparation: Importing Data into Power BI
- Power BI Desktop: Setting up Your Workspace
- Transforming Data Using Power Query Editor
- Establishing Relationships Between Tables
- Creating a Star Schema for Optimal Performance
- Introducing Measures and Calculated Columns
- DAX (Data Analysis Expressions): An Introduction
- Building Your First Semantic Model
- Working with Hierarchies for Detailed Analysis
- Data Refreshing and Maintaining Model Integrity
- Optimizing Model Performance
- Best Practices for Semantic Modeling in Power BI
- Sharing and Publishing Your Semantic Model
- Troubleshooting Common Issues in Power BI Semantic Models
- Key Takeaways and Final Thoughts
- FAQs
1. What is a Semantic Model in Power BI?
A semantic model is a structured layer within Power BI that enables users to define relationships, calculations, and aggregations on imported data, making it more accessible for reporting and analysis. It includes various tables, relationships, and calculations that enhance data understandability and enable users to draw insights from raw data.
2. Why Use Semantic Models?
Semantic models simplify data interpretation for non-technical users, providing a user-friendly structure for data visualization. By setting up a semantic model, Power BI users can:
- Integrate data from different sources
- Create centralized metrics
- Improve data performance in reports
- Facilitate data governance and consistency
3. Understanding Data Modeling Basics in Power BI
Before diving into semantic models, it’s essential to grasp data modeling basics:
- Tables represent data entities.
- Relationships link different tables, allowing cross-referencing.
- Primary and Foreign Keys help establish these connections.
A robust data model lays the groundwork for building effective semantic models.
4. Data Preparation: Importing Data into Power BI
Data preparation is the first step in creating a semantic model. Power BI supports various data sources, from Excel sheets to SQL databases. Importing the data correctly ensures a smoother process when building relationships and visualizations.
- Connect to your data source from the Power BI Desktop.
- Select and load the tables relevant to your analysis.
- Define data types and check for errors during the import to ensure accuracy.
5. Power BI Desktop: Setting up Your Workspace
Power BI Desktop is the primary tool used for creating semantic models. Familiarize yourself with its interface, which includes:
- Report View: Used for creating reports and dashboards.
- Data View: Allows data exploration and manipulation.
- Model View: Where you build relationships and model your data.
6. Transforming Data Using Power Query Editor
The Power Query Editor allows you to clean and transform data before building your model:
- Data Cleaning: Remove duplicates and irrelevant data.
- Data Transformation: Change data formats and values.
- Custom Columns: Create new columns for specific calculations.
This pre-processing step ensures the model will be accurate and optimized for analysis.
7. Establishing Relationships Between Tables
Relationships are the backbone of Power BI’s semantic models:
- One-to-Many: Connects a single row in one table to multiple rows in another, typical for primary-to-foreign key relationships.
- Many-to-Many: Used when tables have multiple overlapping values; requires careful handling.
These relationships allow data to flow between tables, supporting dynamic and interlinked analyses.
8. Creating a Star Schema for Optimal Performance
The star schema is a widely recommended model for Power BI due to its simplicity and performance benefits:
- Fact Table: Stores measurable data (like sales or profits).
- Dimension Tables: Contain descriptive attributes (like time, products, or locations).
This structure minimizes redundancy, improves query performance, and provides a clear path for analysis.
9. Introducing Measures and Calculated Columns
In Power BI, Measures and Calculated Columns are essential for creating calculated data:
- Calculated Columns: Defined at the row level and stored in the table, ideal for static calculations.
- Measures: Calculations that are processed only when used in reports, offering flexibility for aggregations like sum, average, and count.
These tools give you the power to create dynamic data points, enhancing model interactivity.
10. DAX (Data Analysis Expressions): An Introduction
DAX, Power BI’s formula language, allows you to create custom calculations and perform data analysis:
- Basic Functions: SUM, AVERAGE, MIN, MAX.
- Conditional Logic: IF, SWITCH for complex conditions.
- Time Intelligence: Year-to-date (YTD), quarter-to-date (QTD), month-to-date (MTD) calculations.
Mastering DAX is vital for building advanced data models, giving you control over complex calculations.
11. Building Your First Semantic Model
With data, relationships, and basic DAX knowledge, you can now build your first semantic model:
- Define the Tables: Load only the essential tables needed for analysis.
- Create Relationships: Establish clear relationships between these tables.
- Add Measures and Columns: Implement calculated columns and DAX measures.
- Validate the Model: Check for accuracy and ensure all data points interact as expected.
12. Working with Hierarchies for Detailed Analysis
Hierarchies simplify data navigation and provide drill-down capabilities in reports:
- Create hierarchies like Year > Quarter > Month for time-based analysis.
- Geographical Hierarchies: Useful for regional reporting (Country > State > City).
Hierarchies make data more intuitive and improve report navigation.
13. Data Refreshing and Maintaining Model Integrity
Consistency is crucial, especially when data updates frequently:
- Data Refreshing: Set up automatic refreshes in Power BI to keep data up-to-date.
- Validation: Regularly check relationships and calculations to prevent errors.
Frequent data refreshing ensures that reports always reflect the most current information.
14. Optimizing Model Performance
Power BI semantic models must be optimized for performance to handle large datasets efficiently:
- Reduce Column Count: Load only necessary columns.
- Aggregate Data: Limit row counts by summarizing data at higher levels.
- Enable Query Folding: Ensure transformations are executed on the server side, reducing processing time.
These steps make your model faster and more responsive.
15. Best Practices for Semantic Modeling in Power BI
To create reliable models, adhere to these best practices:
- Use Descriptive Naming Conventions: Name tables and columns clearly.
- Avoid Calculated Columns in Large Tables: Use measures instead to improve performance.
- Documentation: Keep notes on relationships and calculations for easy maintenance.
Following these tips helps build clean, efficient models that are easy to manage and scale.
16. Sharing and Publishing Your Semantic Model
Once your model is complete, share it for broader use:
- Publish to Power BI Service: Allow others to access and interact with your reports.
- Security Settings: Set permissions to control data access.
- Embed in Apps: Integrate your reports into other platforms for a seamless user experience.
Publishing makes your insights available to stakeholders, enabling data-driven decision-making.
17. Troubleshooting Common Issues in Power BI Semantic Models
Some issues may arise when building semantic models:
- Incorrect Relationships: Ensure relationships align with data points.
- DAX Errors: Check for syntax errors or mismatched functions in DAX expressions.
- Data Inconsistencies: Regularly review data updates to maintain accuracy.
Understanding common issues allows for quicker troubleshooting and smoother report building.
18. Key Takeaways and Final Thoughts
Mastering Power BI semantic models unlocks the potential of data within an organization. From creating accurate calculations with DAX to structuring data using star schemas, a strong grasp of semantic modeling enhances data-driven insights, empowering better decision-making.
19. FAQs
Q1: What is the purpose of a semantic model in Power BI? A semantic model structures raw data, making it understandable and usable in reports, allowing users to draw meaningful insights.
Q2: What is the difference between a measure and a calculated column? Measures perform calculations dynamically, while calculated columns are pre-defined and stored in tables, affecting storage and performance differently.
Q3: Can I use multiple data sources in a single semantic model? Yes, Power BI supports multiple data sources within one model, enhancing versatility and data integration.
Q4: What is DAX, and why is it important? DAX, or Data Analysis Expressions, is Power BI
For more detailed guidance and in-depth training, visit our training here.